Semantic 3D Reconstruction
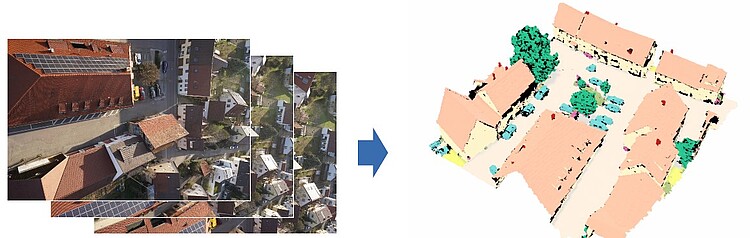
Team: | M. Mehltretter |
Jahr: | 2021 |
Förderung: | Deutsche Forschungsgemeinschaft (DFG) |
Laufzeit: | Since 2021 |
The availability of accurate geospatial information is a prerequisite for many applications, including the fields of mobility and transport as well as environmental and resource protection and typically forms the basis for a comprehensive understanding of an environment of interest. In order to obtain such a comprehensive understanding of a particular environment, it is generally crucial to consider both its geometry and the semantic meaning of the contained entities. One possibility to capture information on both of these aspects simultaneously is the use of image-based methods, i.e., 3D reconstruction and semantic segmentation, while a method that carries out these two tasks jointly, promises to benefit from synergies between them. While first approaches that make use of semantic information to improve dense stereo matching, or vice versa, have been presented in the literature recently, the information flow is commonly only unidirectional, i.e., prior information on one aspect is used to support the estimation of the other aspect, instead of learning both aspects jointly. Moreover, the results are commonly limited to the 2.5D representation of depth maps and are thus rasterised and do not reason about parts of a scene that are occluded in the images.
Addressing these limitations, a novel method based on an implicit function is developed in this project, allowing to estimate a continuous three-dimensional representation of a scene from multi-view stereo images, which encodes the geometry and semantics in a deep implicit field. The basic idea behind this method is to supplement partial observations on the geometry obtained via image matching with learned semantic priors on the shape of objects, allowing to reason about the geometry and semantics also for parts of the scene that are partially occluded in the images. The proposed implicit function is realised as Convolutional Neural Network which allows to learn geometric and semantic priors from training data and is defined in a fully-convolutional manner, meaning that training can be carried out on crops, while large-scale scenes can be reconstructed at test time applying a sliding window-based approach. To investigate the characteristics of the proposed method, simulations on synthetic data as well as experiments on real-world scenes are carried out.
Overall research project: “Integrity and Collaboration in Dynamic Sensor Networks” (i.c.sens)
This project is part of the international Research Training Group “i.c.sens” (https://www.icsens.uni-hannover.de). The aim of the Research Training Group is to investigate concepts for ensuring the integrity of collaborative systems in dynamic sensor networks.