ForstCARe - Forestry Copernicus-based assistance service - Reducing the demand of reference data and fusing sensor data to fill data gaps due to cloud coverage for classification using artificial intelligence (AI)
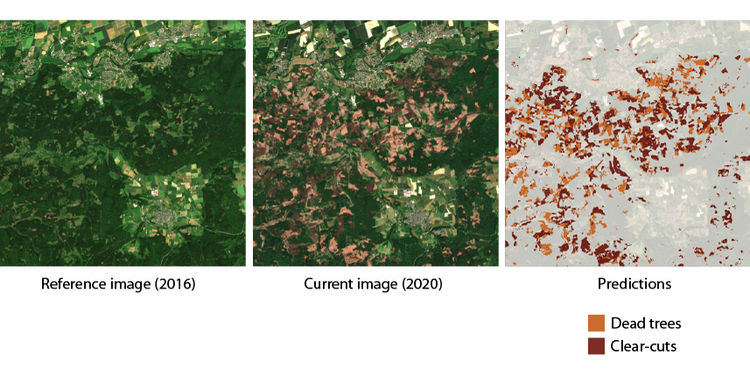
Team: | D.Wittich, F.Rottensteiner, C. Heipke |
Year: | 2021 |
Funding: | Bundesamt für Wirtschaft und Energie |
ForstCARe aims to build a Copernicus-based forestry assistance system by using innovative AI methods, which supports in particular the rapid detection of illegal clearing and the monitoring of novel climate-induced forest damage (drought, storm, pest calamity damage) as well as the monitoring of management measures for reforestation. In all of the applications listed, rapid provision and analysis of data is essential in order to provide timely results on the basis of which illegal clearing can be stopped as quickly as possible or stands infested with pests can be removed as quickly as possible. To this end, AI methods should remove the two currently still decisive obstacles to the widespread and successful use of Copernicus in this application segment:
(a) The lack of representative training data in the necessary quantity and quality. To solve this, novel transfer learning techniques, in particular domain adaptation, and methods that can handle error-prone training data (so-called label noise tolerant methods) will be used. The DA allows the adaptation of a classifier to changing feature distributions in spatial and temporal dimensions. Label noise tolerant methods can detect faulty training data and discard or transform them into a suitable form for further use.
(b) The insufficient timely availability of suitable sensor data due to cloudiness, especially after storms and in the tropics and other cloudy regions. The extent to which the missing optical data from radar data can be simulated using Generative Adversarial Networks (GANs) will be investigated.